Engineering Drawings and Machine Learning
Engineering drawings are a vital part of industries such as construction, manufacturing, and aerospace. These documents serve as blueprints for designing, building, and maintaining complex systems, from buildings and vehicles to electronic components and machinery. Their accuracy and clarity directly impact the success of projects.
However, as valuable as these drawings are, they also present several challenges, particularly when it comes to understanding and accessing the information they contain. In this blog, let’s explore these challenges and understand how machine learning is helping organizations streamline blueprint comprehension and data retrieval.
Common Challenges in Interpreting Engineering Drawings
Understanding engineering drawings requires specific expertise. The process can be time-consuming and prone to error, especially when handled manually. Some of the key difficulties include:
- High Complexity: These drawings often include multiple layers of data, like symbols, annotations, dimensions, and materials, all represented in technical language and visual shorthand. Interpreting them without experience can be overwhelming.
- Limited Domain Knowledge: Not everyone tasked with reviewing these drawings is a domain expert. Years of training and hands-on experience are typically required to read and interpret them accurately.
- Risk of Errors: Manual interpretation increases the risk of mistakes. Misread dimensions, skipped annotations, or misunderstanding of design intent can lead to costly project delays and rework.
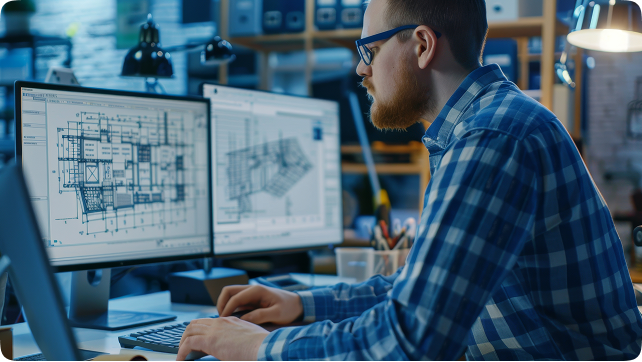
How Machine Learning Enhances Blueprint Comprehension
Machine learning brings a layer of intelligence that simplifies the way teams interact with technical drawings. Its impact can be seen in several areas:
- Automated Information Extraction: ML models can be trained to recognize and extract critical elements such as symbols, text annotations, and measurement data, significantly reducing the reliance on manual data entry.
- Contextual Understanding: Beyond pattern recognition, ML can learn contextual relationships within the drawing. This includes understanding how different components are connected and ensuring compliance with design standards.
- Error Detection: Machine learning can help spot inconsistencies, missing elements, or potential design issues early on. This improves the quality of review processes and helps avoid costly errors later in the project lifecycle.
The Challenge of Searching Engineering Drawings
In many industries, locating a specific engineering drawing or the right information within one remains a significant pain point. This is largely due to:
- Large Volumes of Legacy Documents: Many companies have accumulated decades of engineering documents, often stored in non-standardized or paper-based formats.
- Lack of Metadata: Without structured data like document titles, project tags, or version information, organizing and searching these files is inefficient.
- Manual Search Processes: Traditional search methods depend heavily on individual memory and manual review, making them slow and error-prone.
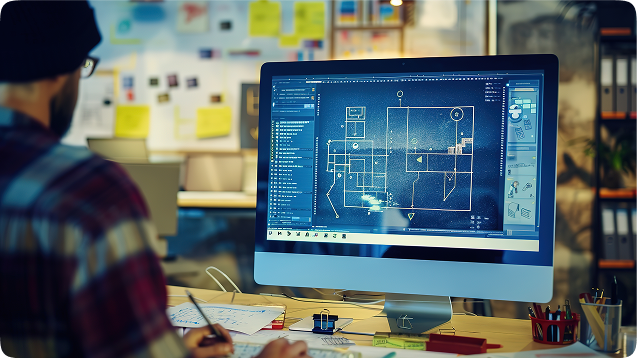
How Machine Learning Improves Search and Retrieval
Machine learning addresses these limitations by introducing automation and intelligence into the search process. Key capabilities include:
- Text and Image Extraction: ML-powered tools can extract both text and graphical elements from scanned blueprints, turning static files into searchable content.
- Visual Element Recognition: By identifying shapes, symbols, and layouts, ML enables users to search based on visual attributes, not just text.
- Automated Metadata Generation: ML models can assign metadata such as file name, project type, or date of creation, improving document indexing and retrieval.
- Advanced Search Functions: Users can search across various parameters, such as text phrases, drawing types, components, or technical specifications, using natural language or visual inputs.
A More Intelligent Way Forward
Machine learning is redefining how organizations interact with their engineering documentation. It reduces the learning curve, lowers the risk of errors, and makes vast archives of technical drawings more accessible. This shift improves operational efficiency and opens the door to more agile, data-driven project execution.
That said, the effectiveness of these ML models, especially for OCR and data extraction, depends heavily on the quality of scanned blueprints.
We specialize in machine learning solutions tailored for engineering and technical document management. Our services help transform static blueprints into intelligent, interactive assets that support better decision-making and faster project execution. If you’re looking to improve the way your organization handles engineering drawings, get in touch with us today. We’d be happy to walk you through the possibilities.
Enhancing your workflow through
AI integration is key to future success.
processes and improve efficiency!

Navin Kumar Parthiban is a seasoned professional in the field of AI technologies and is a Director at iTech India. With a passion for innovation and a keen understanding of the ever-evolving landscape of artificial intelligence, Navin has played a pivotal role in driving iTech India’s success and technological advancements. Navin regularly shares his insights and knowledge through articles, seminars, and workshops. He believes in the power of AI to revolutionize industries and improve people’s lives, and he is dedicated to staying at the forefront of this rapidly evolving field.