Introduction
In the world of engineering, the stakes are high. A small mistake in interpreting a red-line markup or a misinterpreted Single Line Diagram (SLD) can lead to costly delays, compliance issues, or even safety hazards. For years, engineers have relied on manual processes to handle revisions and data extraction from complex documents, often using outdated tools or labour-intensive methods.
Engineering Diagram/Document Handling refers to the process of managing and organizing technical drawings, schematics, and documents within the engineering workflow. This includes tasks like revision tracking, updates, and ensuring accuracy across various design platforms, all of which are crucial for a project’s success. But what if technology could take the burden off their shoulders? What if AI could automatically track revisions, streamline updates, and ensure that all the necessary changes are implemented accurately, without human error?
The good news is that AI is already making this a reality. By automating the tedious and error-prone tasks associated with red-line markups and SLD processing, AI is transforming the way engineers work. In this blog, we’ll explore how AI-powered tools are tackling these challenges head-on and driving efficiency in the engineering industry. From revolutionizing the way, we process revisions to enhancing the accuracy of Single Line Diagrams, AI is helping engineers deliver more reliable and timely results than ever before.
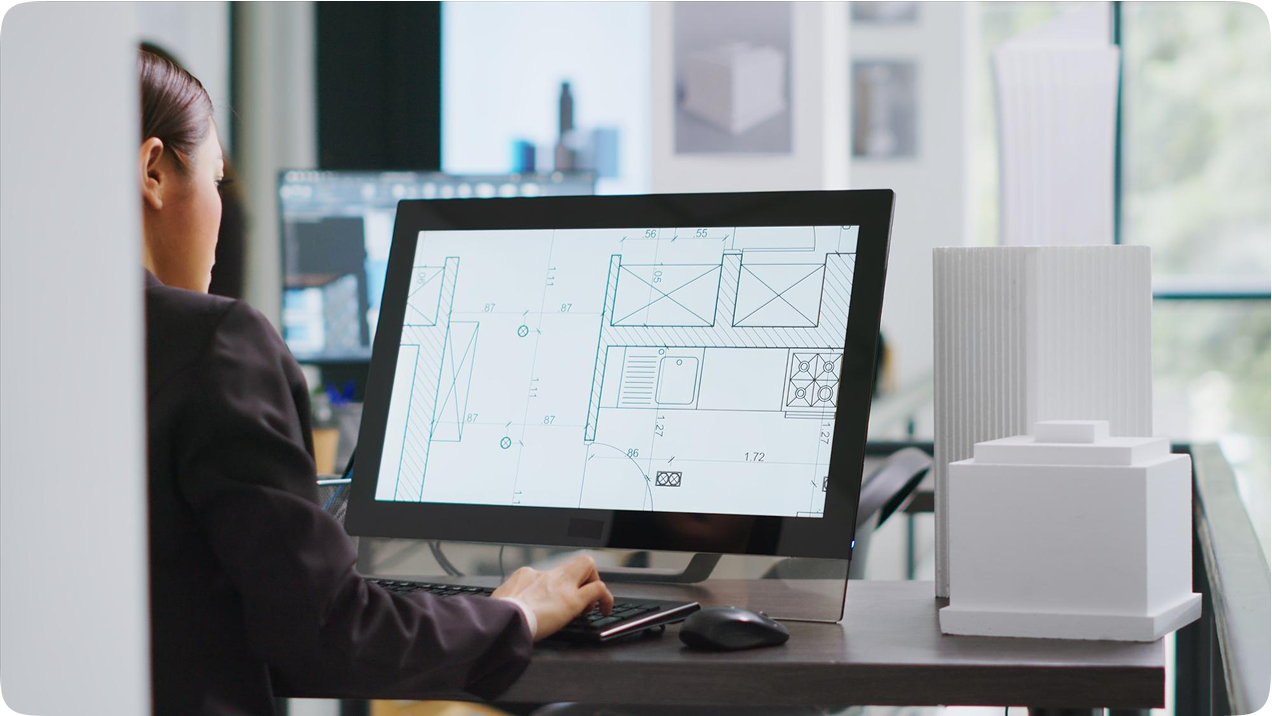
Redline Markups Processing with AI: Enhancing Revision Accuracy
Automated Markup Detection and Digitization
- Traditional red-line markup processing often presents significant challenges, particularly when it comes to extracting hand-drawn annotations or changes from physical or scanned drawings. Engineers are frequently forced to rely on paper-based methods or outdated tools to identify and incorporate revisions, leading to potential errors and inefficiencies.
- AI offers a solution by automating the detection and extraction of red-line markups. Using machine learning algorithms, AI can accurately identify hand-drawn annotations, revisions, and markups from scanned or digital images. This process converts handwritten or graphical revisions into structured digital data, making it easier to integrate changes into CAD models or other engineering design software.
Change Tracking and Version Control
- Managing changes and version control is another critical area where AI significantly boosts efficiency. The manual process of comparing original and marked-up versions of documents is time-consuming and prone to human error. With AI, this process is streamlined and automated, as AI systems can instantly identify and highlight modifications between the original document and its updated version, reducing the chances of missing key changes.
- Additionally, AI helps maintain a continuous version history, which is vital for compliance, audits, and ensuring a transparent workflow. This version control feature ensures that all modifications are validated and correctly implemented, facilitating smoother collaboration among teams while keeping a clear, accurate record of every revision.
Seamless Integration with CAD & Document Management Systems
- AI enhances collaboration by seamlessly integrating with CAD (Computer-Aided Design) systems and document management platforms. AI-powered tools can automatically synchronize red-line revisions with CAD models and engineering drawings, ensuring that changes made on paper or in markup software are accurately reflected in the final CAD files. This eliminates the need for manual updates, reducing the risk of errors and inefficiencies.
- This integration makes it easier for project teams to stay aligned, as they no longer need to manually update CAD drawings with each revision. Instead, the system automatically incorporates changes into the CAD environment, ensuring that the latest version of the design is always accessible and up-to-date.
AI-Powered Handwriting Recognition for Markups
- Handwritten red-line markups often cause confusion and errors in engineering workflows. Deciphering handwritten annotations can be a time-consuming task, and misinterpreting these marks can lead to costly mistakes in the final design. Engineers often spend valuable time reviewing and correcting these handwritten revisions to ensure accuracy.
- AI-powered handwriting recognition algorithms offer a solution by efficiently converting handwritten red-line markups into structured digital text. This process minimizes errors caused by manual interpretation, ensuring that all annotations are accurately captured and implemented into the final design. By automating this task, AI helps engineers save time, reduce mistakes, and streamline the revision process, making it easier to integrate changes into digital design files with confidence.
Automated Classification of Markups
- AI enhances markup processing by automatically classifying red-line annotations into categories such as design modifications, corrections, or comments. This classification helps engineers prioritize changes and understand the significance of each revision.
- By differentiating between markup types, AI speeds up the revision process and ensures that changes are accurately tracked and incorporated into the design workflow. This reduces manual effort, minimizes errors, and ensures that all changes are properly validated and implemented.
Intelligent Overlay and Merge with Existing Drawings
- AI’s ability to overlay red-line markups on CAD drawings in real time allows engineers to compare the marked-up drawing with the original version, helping them quickly identify discrepancies. In many cases, AI can also merge approved changes into the base drawing, ensuring that the final design reflects all modifications in a seamless and accurate manner.
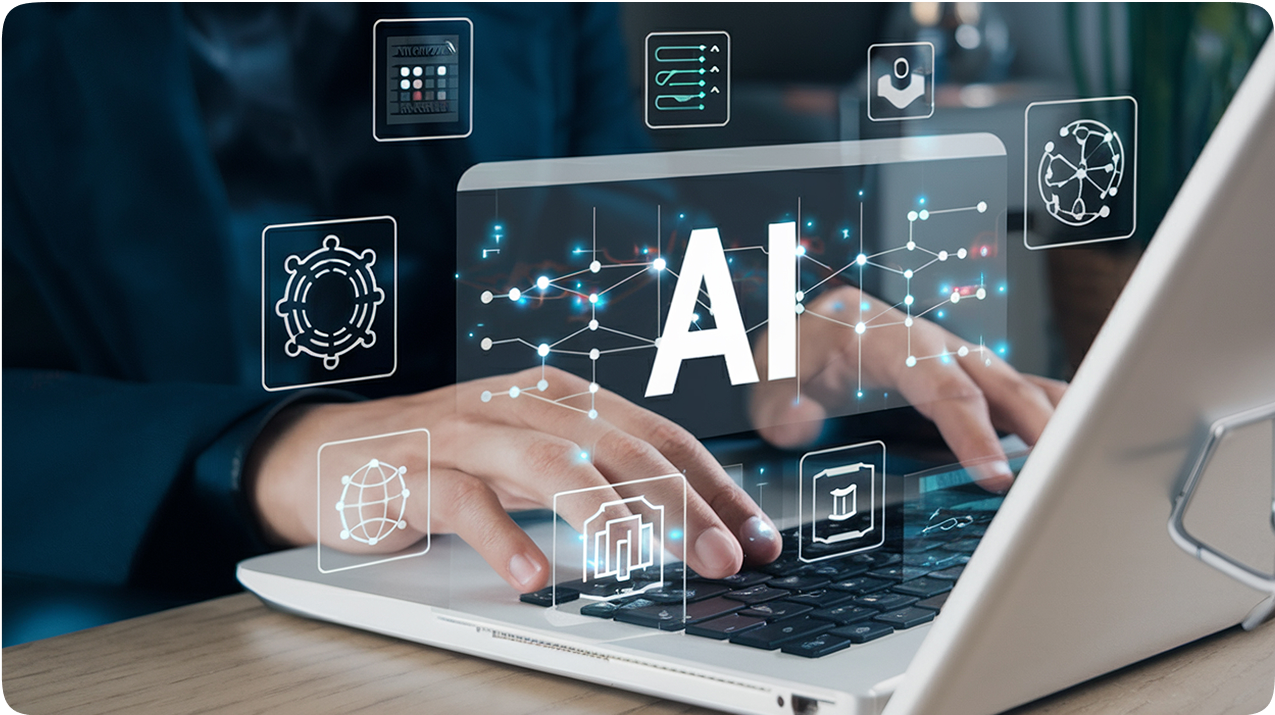
AI-Driven Processing of Single Line Diagrams (SLDs)
Understanding the Significance of SLDs
In electrical engineering, Single Line Diagrams (SLDs) are essential for documenting power distribution systems, electrical grids, and industrial automation. These diagrams simplify the representation of electrical systems, making them vital for system analysis, troubleshooting, and planning. However, manually processing SLDs can be time-consuming and prone to errors. Engineers must extract specific components and data from complex diagrams, often leading to inconsistencies, delays, and integration issues.
Electrical Component Extraction & Data Structuring
AI significantly enhances the processing of SLDs by automating the identification of key electrical components like breakers, transformers, cables, and relays. Using machine learning and image recognition, AI can extract detailed information from SLDs, such as component specifications, cable types, and load details. This data is then converted into a structured format, making it easy to reference for system analysis, planning, or integration into other tools. This automation saves time and reduces the errors associated with manual data extraction.
SLD Conversion for System Integration
In many cases, SLDs need to be converted into different formats for integration with electrical design and simulation tools such as ETAP, AutoCAD, or SKM. AI-driven systems can automatically convert SLDs into industry-standard formats, ensuring seamless integration with other software tools used in the design, analysis, and simulation of electrical systems. This capability eliminates the need for manual conversion, which can be error-prone and time-consuming.
Automated Deliverables Generation
Generating reports and deliverables is an essential aspect of electrical engineering projects, but this process can often be labour-intensive. AI can automate the generation of key deliverables such as:
- Cable Schedules: AI extracts details about cable types, lengths, and connections from SLDs, generating accurate cable schedules for planning and procurement.
- Load Lists: AI maps and validates the connected electrical loads, ensuring that they are accurately captured for analysis and reporting.
- Panel Schedules & Circuit Reports: AI generates structured reports that list panel schedules, circuit configurations, and associated components, aiding in compliance and system analysis.
These automated reports help ensure that all project stakeholders have access to accurate, up-to-date information, reducing errors and delays.
Intelligent Symbol Recognition & Classification
SLDs often use standardized symbols to represent electrical components, but these symbols may vary across different drawings or sources. AI can recognize and classify these symbols, ensuring consistency across SLDs. This recognition helps standardize the symbols used in the diagrams and ensures that they are accurately interpreted across various engineering platforms.
Key Benefits of AI in Engineering Workflow
- Increased Accuracy: AI minimizes human error, making it easier to track revisions and accurately interpret complex documents.
- Time Savings: By automating tasks like markup processing and component extraction, engineers can focus more on creative and strategic aspects of their work.
- Improved Collaboration: AI automatically syncs with CAD systems, ensuring that everyone on the team is working with the most current version of design files.
- Cost Efficiency: By streamlining workflows and speeding up project completion, AI helps reduce the costs associated with delays and errors.
Conclusion
AI-driven automation is transforming how engineers handle red-line markups and Single Line Diagrams (SLDs), making workflows faster, more accurate, and more efficient. A study by McKinsey found that AI could boost productivity in engineering by up to 30% by automating repetitive tasks and reducing errors. By automating revision processing, detecting handwritten annotations, and integrating with CAD and document management systems, AI reduces manual effort and the risk of human error. Additionally, AI processes and structures data from SLDs, converts diagrams into industry-standard formats, and generates automated deliverables, saving time and resources for engineers.
The future of engineering is closely tied to AI. As AI tools improve, they will continue to automate more tasks, making processes even faster and more accurate. In fact, research suggests AI could save the engineering industry billions of dollars annually. Looking ahead, AI will not only help engineers work more efficiently but also unlock new possibilities, such as predicting failures, optimizing designs, and enhancing collaboration across teams.
Ready to embrace the future of engineering?
Contact us today to see how our AI-driven solutions can help you improve your workflows and deliver better results faster!
Enhancing your workflow through
AI integration is key to future success.
processes and improve efficiency!

He is a seasoned machine learning engineer with a wealth of hands-on experience .Pravin Kumar has a strong foundation in OCR, computer vision, and deep learning and leads the ML team at iTech India. He is an expert in a diverse range of programming languages and frameworks, including Python, CPP, Scala, JS, and React, and has a deep understanding of machine learning algorithms and techniques. He and his team have broken new ground in a wide array of projects spanning image recognition, object detection, and text extraction. This has enabled him to tackle complex projects and deliver top-tier results for real-world challenges.